How AI is revealing the universe's original 'settings'
Astronomers used artificial intelligence to calculate the five cosmological parameters that describe the entire universe in computer simulations with unprecedented precision.
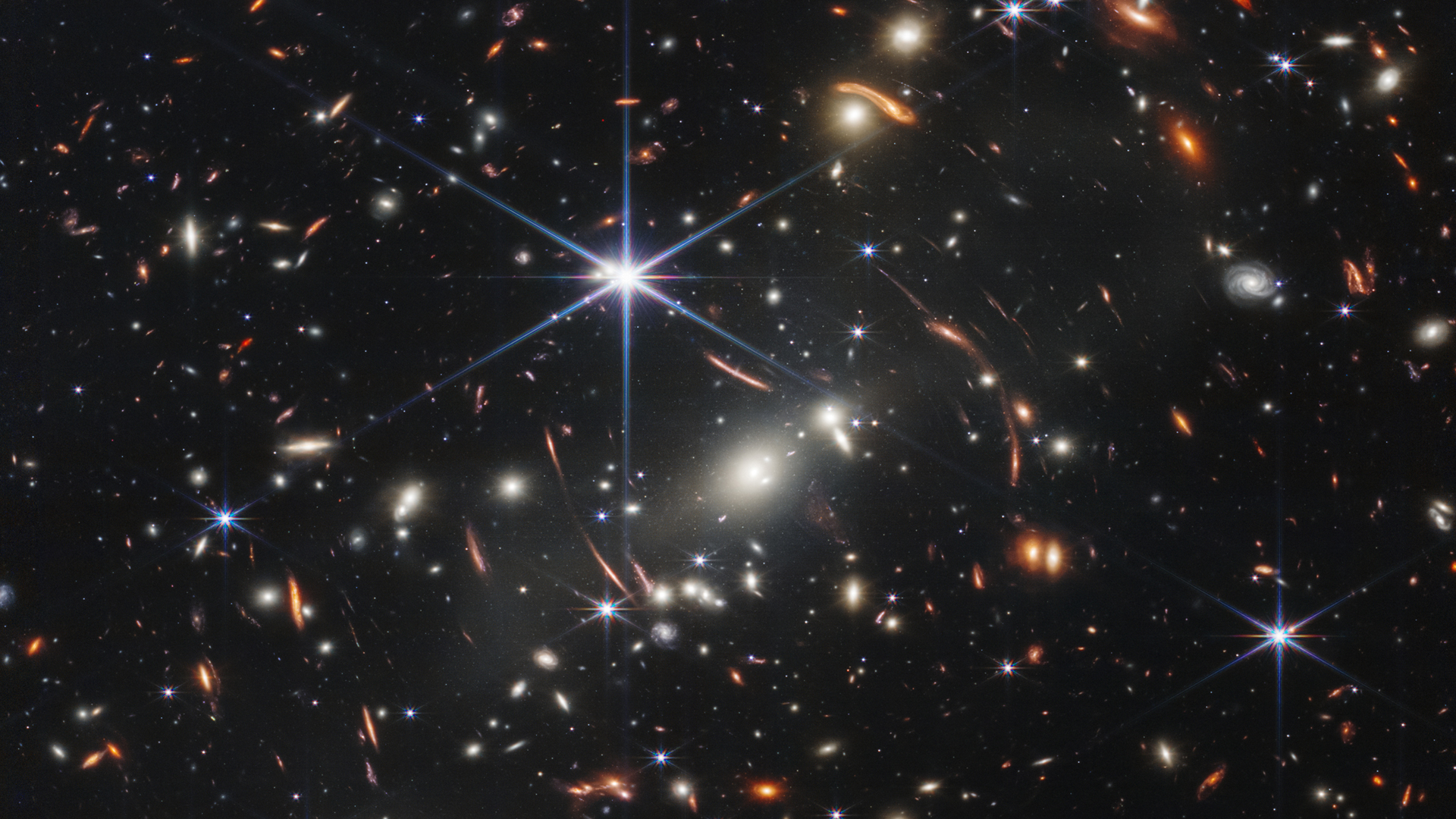
Artificial intelligence is all the rage these days. When we think of AI, we're usually thinking of uncanny "deepfake" images and Chat GPT's written responses — but astronomers are actually using it to make significant discoveries, too.
Researchers from the Flatiron Institute's Center for Computational Astrophysics (CCA) in New York City recently used AI to determine five cosmological parameters — the numbers that describe the entire universe in astronomers' computer simulations — with unprecedented precision.
These parameters can be thought of as the "settings of the universe that determine how it operates on the largest scales," said astronomer Liam Parker, a co-author on the new work, in a statement.
With their AI helper, the team extracted these parameters from a dataset containing information on over 100,000 galaxies observed as part of the expansive Sloan Digital Sky Survey (SDSS). Massive surveys like SDSS are critical to understanding the universe as a whole; by looking at how galaxies are clustered across space, astronomers can narrow down the values of parameters describing how much dark matter is in the universe, what the universe was like right after the Big Bang, and more.
"Each of these surveys costs hundreds of millions to billions of dollars," said co-author and CCA astronomer Shirley Ho in the release. "The main reason these surveys exist is because we want to understand these cosmological parameters better. So if you think about it in a very practical sense, these parameters are worth tens of millions of dollars each. You want the best analysis you can to extract as much knowledge out of these surveys as possible and push the boundaries of our understanding of the universe."
With a big survey comes a big amount of data, and astronomers have been brainstorming how to get the most information out of that mountain of information. The CCA team's approach used AI to analyze small-scale details in the distribution of galaxies in the universe—something that had never been done before, as past work only focused on larger-scale trends.
"For a couple of years now, we've known that there's additional information there; we just didn't have a good way of extracting it," said Princeton astronomer and lead author Chang Hoon Hahn in the release.
Get the Space.com Newsletter
Breaking space news, the latest updates on rocket launches, skywatching events and more!
To make an AI model worth using, you first need to train it on what to look for — somewhat like training a budding astronomy student to develop a good intuition for physics, where they're able to recognize patterns in their problem sets.
For this training, Hahn and collaborators generated 2,000 simulated universes, each with different cosmological settings. Importantly, they knew what cosmological values were used in each of these simulations, so the AI knew what the correct answer was. The simulations also included real-life challenges encountered in galaxy surveys, like blurring from the atmosphere or an imperfect telescope mirror, to make sure the AI's training was realistic.
Once the model graduated from its training bootcamp, the team fed it real data from the SDSS Baryon Oscillation Spectroscopic Survey, and the results were stunning. For example, the AI method determined the parameter describing the universe's "clumpiness" with less than half the uncertainty of traditional methods.
This method was "equivalent to a traditional analysis using around four times as many galaxies" according to the statement, making it possible for astronomers to do more with less data and push the boundaries of what's possible.
And AI was critical to making this possible. "If you didn't have the machine learning, you'd need hundreds of thousands" of simulations, a practically intractable number, Hahn said in the release.
A better knowledge of the universe's fundamental settings could even help resolve a big cosmic mystery: The Hubble tension, where different experiments estimate different values for the Hubble constant, which describes how fast the universe is expanding. With new surveys like the European Euclid survey coming online, methods like this AI-powered algorithm will be critical to making the most of that new data.
This study was published Aug. 21 in Nature Astronomy.
Join our Space Forums to keep talking space on the latest missions, night sky and more! And if you have a news tip, correction or comment, let us know at: community@space.com.
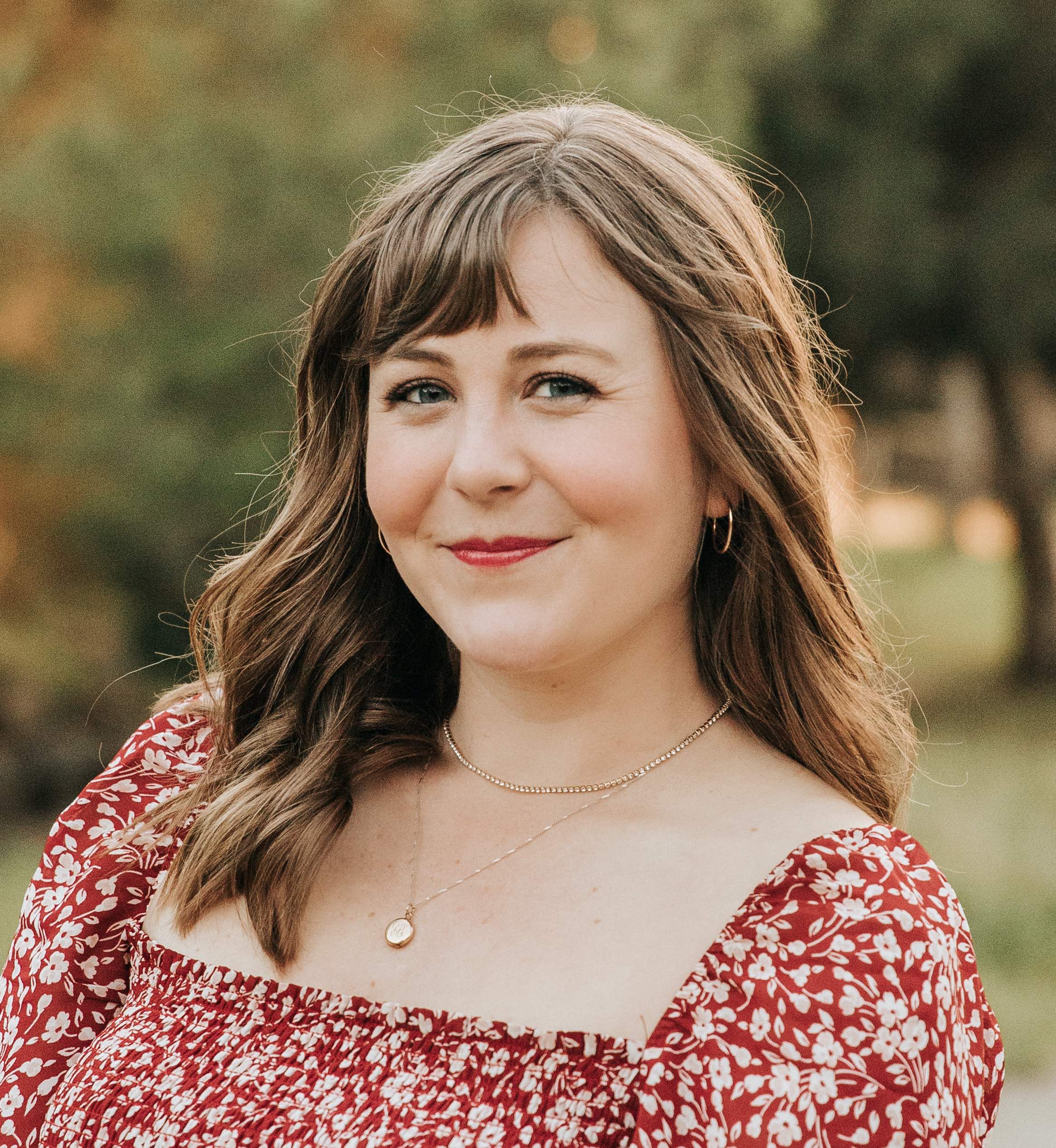
Briley Lewis (she/her) is a freelance science writer and Ph.D. Candidate/NSF Fellow at the University of California, Los Angeles studying Astronomy & Astrophysics. Follow her on Twitter @briles_34 or visit her website www.briley-lewis.com.
-
Torbjorn Larsson They improve on the BOSS survey but else there are no surprises, they are consistent with earlier surveys. And as in most such cases they prefer a Hubble constant that is close to the Friedmann et al value, H_0 ~ 69 km*s^-1*Mpc^-1.Reply -
Gibsense
Confirming 'no surprise' is of course important but for a layman the above gives a Hubble Constant of.... ?Torbjorn Larsson said:he Friedmann et al value, H_0 ~ 69 km*s^-1*Mpc^-1.