Predicting future floods: New AI tool gives realistic satellite-like views
'One day, we could use this before a hurricane, where it provides an additional visualization layer for the public.'
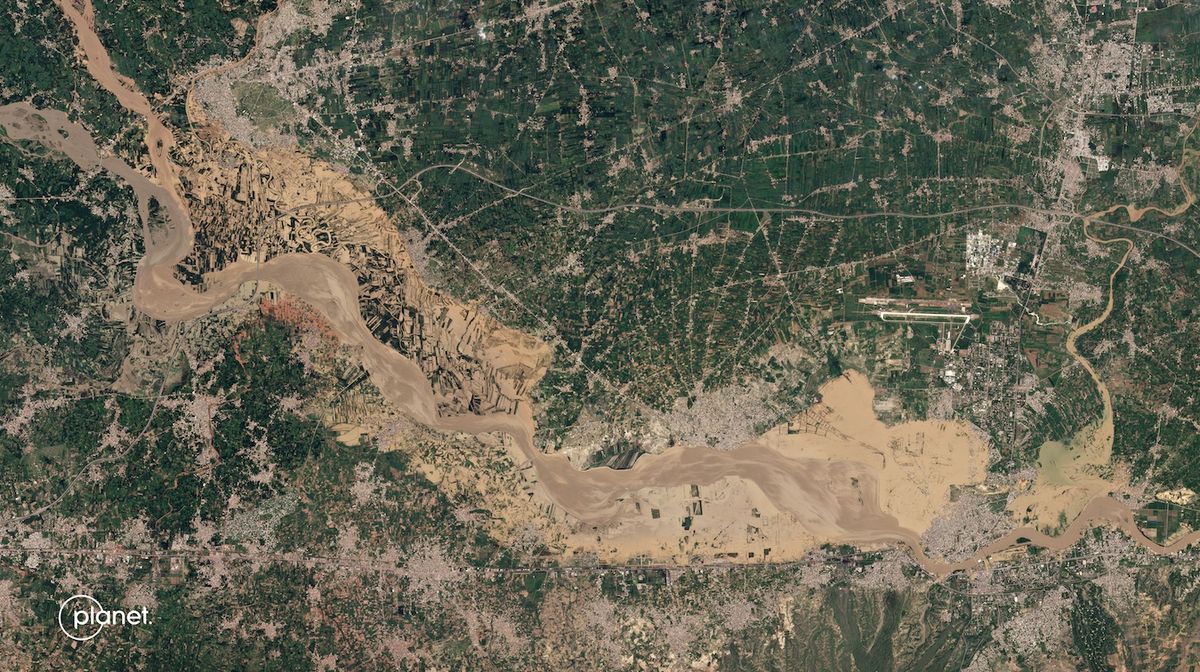
MIT scientists are developing an artificial intelligence (AI) tool that creates realistic satellite images of potential flooding scenarios.
The tool combines a generative AI model with a physics-based flood model to predict areas at risk of flooding and then generate detailed, bird's-eye-view images of how the region might look after the flood, based on the strength of an approaching storm.
"The idea is, one day, we could use this before a hurricane, where it provides an additional visualization layer for the public," Björn Lütjens, a postdoc in the Department of Earth, Atmospheric and Planetary Sciences at the Massachusetts Institute of Technology (MIT), said in a statement.
"One of the biggest challenges is encouraging people to evacuate when they are at risk," added Lütjens, who led the research while he was a doctoral student in MIT's Department of Aeronautics and Astronautics (AeroAstro). "Maybe this could be another visualization to help increase that readiness."
Related: Safety first: NASA pledges to use AI carefully and responsibly
The team trained a machine learning model called a conditional generative adversarial network, or GAN for short, which creates realistic images using two neural networks working against each other.
The first network, called the "generator," learns by studying real examples, like satellite images of areas before and after a hurricane. The second network, the "discriminator," acts as a critic, trying to tell apart the real images from the fake ones created by the generator. Together, they improve until the generated images look convincingly realistic.
Get the Space.com Newsletter
Breaking space news, the latest updates on rocket launches, skywatching events and more!
Each network learns and improves automatically based on feedback from the other. This back-and-forth process aims to create synthetic images that are nearly identical to real ones.
However, GANs sometimes produce "hallucinations" — features in the images that look real but are factually incorrect or shouldn't be there.
"Hallucinations can mislead viewers," said Lütjens. "We were thinking: How can we use these generative AI models in a climate-impact setting, where having trusted data sources is so important?"
That's where the physics model comes in.
To demonstrate their model's credibility, the researchers applied it to a scenario for Houston, generating satellite images of flooding in the city following a storm comparable in strength to Hurricane Harvey, which actually hit in 2017. They then compared their AI-generated images to actual satellite images, as well as images created without the assistance of the physics-flood model.
Not surprisingly, without the aid of the physics model, the AI images were highly inaccurate, with numerous "hallucinations" — specifically, the images depicting flooding in regions where it would not be physically possible. But the physics-reinforced method's images were comparable to the real-world scenario.
The scientists envision that this tech should be most applicable to predicting the outcomes of future flooding scenarios by producing trustworthy visuals to help policymakers better prepare for and make informed decisions about flood planning, evacuation and mitigation efforts.
In their press release, the scientists say that policymakers typically gauge where flooding might occur based on visualizations in the form of color-coded maps.
"The question is: Can visualizations of satellite imagery add another level to this, that is a bit more tangible and emotionally engaging than a color-coded map of reds, yellows and blues, while still being trustworthy?" Lütjens said.
This is an important example of how space-based technology can help in managing the unfolding climate crisis, which is making extreme events, like flooding and hurricanes, more likely.
The team's method is still in the proof-of-concept stage and needs more time to "study" other regions to be able to predict the outcomes of different storms. This will require further training on many more real-world scenarios.
"We show a tangible way to combine machine learning with physics for a use case that's risk-sensitive, which requires us to analyze the complexity of Earth's systems and project future actions and possible scenarios to keep people out of harm's way," said Dava Newman, professor of AeroAstro and director of the MIT Media Lab. "We can't wait to get our generative AI tools into the hands of decision-makers at the local community level, which could make a significant difference and perhaps save lives."
The team published their work last month in the journal IEEE Transactions on Geoscience and Remote Sensing.
Join our Space Forums to keep talking space on the latest missions, night sky and more! And if you have a news tip, correction or comment, let us know at: community@space.com.
A chemist turned science writer, Victoria Corless completed her Ph.D. in organic synthesis at the University of Toronto and, ever the cliché, realized lab work was not something she wanted to do for the rest of her days. After dabbling in science writing and a brief stint as a medical writer, Victoria joined Wiley’s Advanced Science News where she works as an editor and writer. On the side, she freelances for various outlets, including Research2Reality and Chemistry World.